Research links cell signaling, location and patient prognosis in brain tumors
Rebecca Ihrie, PhD, is Associate Professor of Cell and Developmental Biology at Vanderbilt University. Ihrie is interested in understanding these heterogeneous mixtures of cells and how they vary across patients.
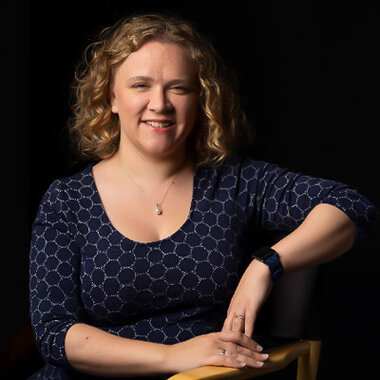
The Ihrie Lab at Vanderbilt develops new approach to associate cell type to overall survival
Sometimes the smallest things can help you see the bigger picture. That’s the idea behind one professor’s research into brain cancer. By revealing the abnormal cells that are most aggressive within brain tumors, we can find answers about which cell features may lead to which clinical outcomes.
Rebecca Ihrie, PhD, Associate Professor of Cell and Developmental Biology at Vanderbilt University, recently talked with us about glioblastoma, one of the most common and malignant forms of primary brain tumor.
Although glioblastoma has been a heavy focus of study for many years, prognosis has not improved much in recent decades. Current standard treatments center on the alkylating agent temozolomide in addition to radiotherapy. While use of this agent extends survival to some extent, overall prognosis and survival outcomes remain poor.
Mass cytometry is a primary research approach used in the Ihrie Lab, being particularly informative for glioblastoma investigation. “When we think about everything going on within a cell, DNA and RNA profiling, which have been widely used in brain tumors and many other tissues, are certainly very informative. But when we think about the functional repertoire of a cell, this information is incomplete,” explains Ihrie. “Mass cytometry allows us to complement what we have learned at the DNA and RNA level to better understand beyond copy number analysis, sequence analysis and transcript analysis how cells are dynamically responding to their neighbors and what their signaling capability at the protein level is.”
The complexity of glioblastoma is evident within tumors, which can contain multiple cancer lineage cells resembling different stages of neural stem cell differentiation; neurons, astrocytes, and glia (the resident cells of the brain); various immune cells; and endothelial cells. Three major subtypes of glioblastoma have been classified, distinguished primarily by alterations in growth factor receptor gene copy number as well as transcriptional signatures that resemble different progenitor cells within the brain.
Ihrie is interested in understanding these heterogeneous mixtures of cells and how they vary across patients. She discusses two recent questions her lab and collaborators are currently focused on: How can we identify highly malignant phenotypes across glioblastoma, finding the most aggressive cells that drive recurrence but that might be rare and variable across patients? And how can we do discovery work in smaller cohorts of patients? “We really wanted to leverage all of the information generated from approaches like mass cytometry, where we can obtain millions of cells and lots of data on specific parameters to figure out which of these parameters can help us find the cells that are driving the worst outcomes and then identify their targetable features,” Ihrie says.
Mass cytometry allows us to complement what we have learned at the DNA and RNA level to better understand … how cells are dynamically responding to their neighbors and what their signaling capability at the protein level is. ”
– Rebecca Ihrie, PhD
Stratifying patients with RAPID
Risk Assessment Population IDentification (RAPID), an unsupervised and automated machine learning algorithm, identifies phenotypically distinct cell populations and determines whether these populations stratify patient survival. The algorithm couples the per-cell data obtained using mass cytometry to continuous features like progression-free survival, measured in days or weeks, and overall survival, which in glioblastoma has a median of about 15 months.
Initially, the team analyzed over 2 million glioblastoma cells from 28 patients by mass cytometry, using a panel of 40 different parameters to find immune, endothelial and other infiltrating cells within brain tumors.
The team assigned all cells to clusters based on phenotype and determined which clusters were meaningfully associated with a better or worse outcome. RAPID analysis identified both glioblastoma-negative prognostic (GNP) cells, whose abundance is predictive of especially bad outcome, and glioblastoma-positive prognostic (GPP) cells, whose abundance indicates a long overall survival. She notes that since these survival differences had not been discovered through other data methods at the genomic DNA or RNA level, “seeing something that stratified survival was very exciting, because it gives us an opportunity to better understand the biology of these tumors and think about how to target them.”
The in-depth data gleaned from their mass cytometry experiments revealed distinct and mixed phenotypes with opposing expression patterns that further distinguished the two prognostic groups. “Access to the high-dimensional data from CyTOF® was fantastic for us to be able to do additional ongoing discovery work in these tumors and to understand these phenotypes,” adds Ihrie. “But we want to be able to translate this to approaches that neuropathologists typically use.”
Translating CyTOF findings to neuropathology
Ihrie wondered if brain tumor location could affect cancer cells and immune cells within the tumor. The goal was to apply the phenotypes discovered from suspension mass cytometry and pinpoint their location within individual tumors or across tumor classifications.
Based on MRI images, the team asked whether glioblastoma tumors contacting specific structures within the brain have better or worse outcomes. At initial diagnosis, about 50% of patients had a tumor that contacts the lateral ventricles and the stem cell niche that resides there. These patients had poorer overall survival. A smaller subset had a tumor that contacts the other major stem cell niche of the brain, the dentate gyrus—but surprisingly, contact with this niche did not correlate with survival differences.
Overlaying their originally determined cell phenotypes with tumor location classification, they found a cluster of cells that is enriched in noncontacting tumors with protein expression levels very closely resembling the glioma-positive prognostic phenotype.
Other known predictors of outcome, such as the size of the tumor, the ability of the surgeon to resect it and bulk DNA and transcriptomic profiles, did not explain the effects of ventricular contact and raised novel questions about the biology behind these differences in outcome. Moving forward, Ihrie is interested in coupling MRI images, a routine visualization for glioblastoma patients, to this type of information to help decide on treatment options.
Connecting the dots on the influence of tumor location on potential tumor prognosis
One challenge with traditional imaging approaches is the inability to view and analyze all parameters simultaneously. “Wouldn't it be nice if we could measure everything together? That's something we are working on now, together with the Bursky Center for Human Immunology & Immunotherapy at Washington University in St. Louis using Imaging Mass Cytometry™,” Ihrie says. “We are eager to take all the parameters we used on dissociated tumors in suspension and apply them across the tissue to get more information, including spatial data for better classification of patients.”
Ihrie next used Imaging Mass Cytometry to identify and associate cell-to-cell variation and distribution within different tumors to phenotypes. The team developed and validated a brain-centered imaging panel that included lineage-specific transcription factors, phosphoproteins and other cancer-centric markers that overlap with the panel used for earlier RAPID studies.
In glioblastoma samples, images revealed a nonrandom distribution of signaling, with separate clusters of cells signaling together. Further study could isolate these signaling groups to decipher their role in the tumor and whether distance from the stem cell niche might come into play.
Particularly in glioblastoma, where transcriptomic and other genomic analyses have not readily allowed stratification of patients into different groups based on outcome, mass cytometry measurements at the protein level that include phosphorylation events have helped classify prognostic subgroups. The custom workflow, RAPID, is now published, and it generates simple stratification assays that use high-dimensional data to compare findings in fixed tissue and suspension.
“Ultimately, we are very excited to find that stem cell niche contact changes the cancer cell and immune cell features and is linked to some of the differences in prognosis that we found before. And importantly, these phenotypes are not uniformly distributed through the tissue. The ability to go back and forth between what we can discover in suspension and in fixed tissue has been an incredibly useful tool for us,” concludes Ihrie.
Important links:
- Watch Webinar: Linking cellular signaling, location and patient prognosis in brain tumors | Rebecca Ihrie, PhD
- Download Article: Research links cell signaling, location and patient prognosis in brain tumors | Rebecca Ihrie, PhD
References:
- Leelatian, N., Sinnaeve, J., Mistry, A.M. et al. “Unsupervised machine learning reveals risk stratifying glioblastoma tumor cells.” eLife 9 (2020): e56879.
- Mistry, A.M., Hale, A.T., Chambless, L.B. et al. “Influence of glioblastoma contact with the lateral ventricle on survival: a meta-analysis.” Journal of Neuro-oncology 131 (2017): 125–133.
- Hakobyan, S. et al. “Complement biomarkers as predictors of disease progression in Alzheimer’s disease.” Journal of Alzheimer's Disease 54 (2016): 707–716
- Mistry, A.M., Wooten, D.J., Davis, L.T. et al. “Ventricular-subventricular zone contact by glioblastoma is not associated with molecular signatures in bulk tumor data.” Scientific Reports 9 (2019): 1842.
For Research Use Only. Not for use in diagnostic procedures. Patent and License Information: www.standardbio.com/legal/notices. Trademarks: www.standardbio.com/legal/trademarks. Any other trademarks are the sole property of their respective owners. ©2025 Standard BioTools Inc. All rights reserved.